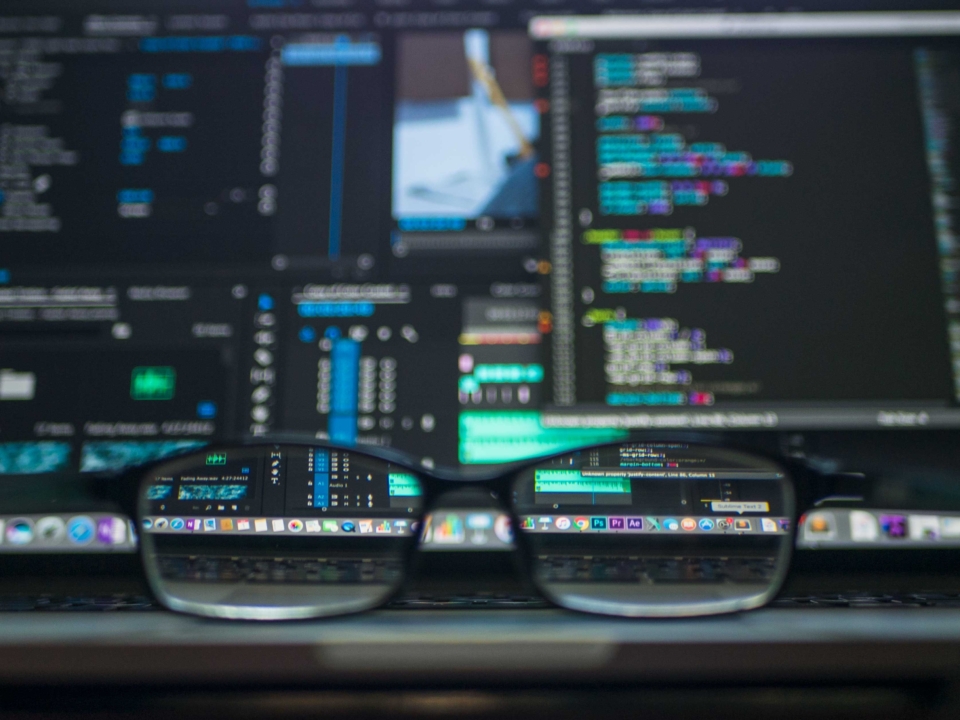
Process intelligence allows organizations to improve their processes, increasing their operational quality across the board. Business leaders are thus willing to adopt a variety of tools to achieve this goal. Those tools are known by various names, such as process intelligence and process mining software.
While these tools have distinct capabilities, they also share many features, creating debate among business analysts about the best ways to implement process improvement initiatives.
This guide discusses the power and benefits of process intelligence, its implementation, examples, and future insights.
Unlock better data and cost savings with the Definitive Guide to Business Process Automation
What is process intelligence?
Process intelligence is the use of methods and tools — usually in the form of software – to provide greater control over process management. Its primary goal is to improve operational efficiency, often by removing bottlenecks.
Process analysts also view process intelligence as the practice of collecting and analyzing data for the purposes of identifying bottlenecks and other barriers to process excellence.
Process intelligence vs. traditional process management
The components of traditional process management consist of process mapping, process mining, and process discovery. Process mapping relies on teams of consultants tracking a process from beginning to end by closely monitoring the actions workers perform to complete the process.
Process mining uses backend software to record workflows step-by-step, based on user interaction with systems. Process discovery is a more recent alternative to process mining that tracks workflows via user interfaces, although it still requires manual evaluation of the results.
However, these traditional methods provide an incomplete view of an organization’s processes and how they are executed. Furthermore, manually monitoring processes can alter the ways in which workers perform those processes due to their awareness of being observed.
Even the automatic capture of log files misses manual steps and other steps that occur outside the application. In contrast, process intelligence automatically and continuously acquires data at scale, resulting in clear visibility into an organization’s journey towards improving its processes.
Reading recommendation: What Is Workflow Management?
Components of process intelligence
Components of process intelligence include the following:
- Data collection and integration
- Process mining
- Process modeling
- Analytics and visualization
- Digital twinning
These activities often use machine learning (ML) algorithms to collect and analyze data from enterprise solutions like enterprise resource planning (ERP) and customer relationship management (CRM). These tools may work with both historical and real-time data.
Data collection and integration
Process intelligence collects data from various sources, including company databases and other IT systems. They may also use employee input to obtain a comprehensive understanding of the organization’s processes.
Process mining
Businesses often consider their processes to be simple workflows, but they’re usually more complicated than this due to deviations, iterations, and interactions between processes. Process mining monitors the current condition of processes rather than their ideal state.
For example, it uses real-time data to generate incident reports that can identify bottlenecks or eliminate unnecessary steps. These factual insights allow organizations to better direct their efforts towards improvement.
Process modeling
Process modeling is a set of practices that allow designers to visualize a business’s workflows and processes. It involves consideration of each step in a process, providing a thorough understanding of the activities and tasks comprising that process.
Important factors in process modeling include a workflow’s activities and events, including timelines. The people involved are also crucial to process modeling. In addition, designers examine decision points, along with their paths and possible outcomes.
Analytics and visualization
The graphical representation of a process model helps analysts improve process efficiency.
Advanced visualizations include dashboards and data tables, which analysts scrutinize carefully and export into other tools like business intelligence (BI) solutions and spreadsheets.
Digital twinning
A digital twin of an organization (DTO) is a virtual model of a process. It’s often used to study aspects of the process, such as goals and performance, as indicated by key performance indicators (KPIs).
DTOs are also useful for a detailed analysis of the process at the transaction level. Optimizing performance with a DTO requires regular updates regarding product quality, resource utilization, and meeting customer satisfaction levels.
Benefits of process intelligence
Process intelligence provides a systematic, data-driven means of improving processes, generally by identifying bottlenecks and automation opportunities. Specific benefits include the following:
- Improved efficiency,
- Enhanced decision-making,
- Compliance and risk management,
- Continuous improvement,
- Cost reduction, and
- End-to-end process visualization.
Improved process efficiency and effectiveness
Process intelligence tools can help analysts automate processes, thus improving operational efficiency. They can obtain results and implement changes faster than they could using manual intervention, which also reduces the risk of human error in addition to saving time. Achieving these results requires effective collaboration between departments as the solution gathers data, maps it, and analyzes processes.
Business leaders must therefore understand that process improvement is a long-term project requiring a sustained effort when deciding to implement such an initiative.
Enhanced decision-making
Modern organizations generate a great deal of data in their daily operations through both digital systems like SAP and physical systems like IoT sensors. Process intelligence solutions allow them to identify patterns in their processes, which can provide insight into making decisions. These solutions can also provide this data to a centralized location, allowing users to view data from a single dashboard.
Compliance and risk management
Process intelligence helps organizations remain compliant with government regulations and industry standards, identifying areas where processes are at risk of non-compliance, and alerting organizations to those issues before they become critical.
Overall quality improvements that accompany process intelligence initiatives further reduce the risks of non-compliance.
Continuous improvement
Process improvement solutions can identify the tasks that are most suitable for automation. They use task mining to analyze processes, including their impact on business outcomes, complexity and frequency.
These results, coupled with an emphasis on automation efforts, allow businesses to re-build processes with a structured approach to improvement. Process intelligence tools are implemented with the knowledge that the time for improvement comes before workflows stop running smoothly.
The continuous, intentional data analysis they perform identifies both discrepancies and patterns, thereby providing continuous process improvement.
Cost reduction
Process intelligence tools reduce the cost of running processes first by streamlining them. Breaking processes down into parts can reveal steps that can be automated — and sometimes eliminated altogether. Cutting out this process waste is the best way to reduce production costs.
Deviations, bottlenecks, and silos can also be identified by process intelligence solutions. When these blockers are eliminated, operating expenses decrease, allowing leaders to allocate a larger budget to other areas of business.
End-to-end process visualization
Process intelligence begins with an overview of each process and all it entails, from beginning to end. After all, no process can be optimized until it is broken down into steps. First, waste is identified and eliminated, then automation is applied to appropriate steps. After a few rounds of testing and adjustments, the improved, fully visualized process can be implemented.
How to implement process intelligence
Process intelligence isn’t an exercise that you can perform only once. Success in this endeavor requires consistent review and change management of the following phases:
- Define goals and objectives
- Select appropriate process intelligence tools
- Prepare and integrate data
- Mine and analyze processes
- Apply insights to optimize processes
1. Define goals and objectives
The first step in implementing process intelligence is to establish clear goals and objectives, such as cost reduction, greater customer satisfaction, improved compliance, or increased efficiency. These goals should be quantitative and measurable, with forethought given to how success or failure will be measured.
2. Select appropriate process intelligence tools
Many process intelligence solutions are currently available, which can be overwhelming when choosing one for the first time. Your choice may be simplified by sorting the options into broad categories.
These categories include process analytics that run on existing BI platforms, such as Microsoft PowerBI, Qlik Sense, and Tableau. Task mining software is another major category, which collects and processes information from workstations for the purpose of improving processes.
In comparison, process mining tools extract useful data from sources like event logs. Hybrid solutions that perform both process mining and task mining also exist. You may also opt for process discovery software, which performs the discovery phase for process and task mining.
3. Prepare and integrate data
Like most technology solutions, implementing a process intelligence tool involves combining data from several different sources into one platform. Before this takes place, however, data must first be extracted, cleansed, transformed, and deposited onto a master server. The master server then acts as a messenger between the human requesting data and the data sources.
The insights business leaders gain from contextualized data can help them quickly determine the actions they need to take. These insights can lead to innovations that improve operational efficiency and leverage opportunities for expansion.
4. Mine and analyze processes
Many tools for process mining and analytics exist, from completely automated process discovery solutions to self-built process analytics. However, they generally follow the extract-transform-load (ETL) workflow that’s already familiar to many BI analysts.
This workflow organizes and processes the raw data required for advanced analytics.
The extract phase collects data from enterprise resource systems or directly from the employees who perform the tasks. The transformation phase processes this data and transforms it as needed for analysis, which typically includes cleaning and de-duplicating. The load phase moves the data to a database or warehouse where users can visualize and analyze it from dashboards.
5. Apply insights to optimize processes
At this point in the implementation, you have a complete process model that describes how employees work and the general steps they perform. The data is validated and stakeholders agree that the model accurately describes the real processes.
The next step is to capture the context of the data as completely as possible, providing a more useful view of the data. This context allows analysts to relate workflows and their timings to the complete set of conditions for that process. The insight that contextualized data provides is invaluable for developing the actions needed to improve processes.
Common challenges
Common challenges to implementing process intelligence may be classified into:
- Personnel issues
- Implementation strategies
- Prioritizing the processes to improve
Personnel Issues
As of 2023, employees with expertise in process intelligence are in very short supply. Most organizations lack in-house personnel with these skills, requiring them to outsource.
It’s important to ensure that these experts also have advanced knowledge in related areas, such as analytics, sustainable data and ethical AI development. These partnerships should fill specific talent gaps rather than completely replacing in-house teams.
Implementation strategies
The complexity of implementing process intelligence is largely due to the many components that must work well together, including AI, analytics, and automation. However, business purposes are comparatively easy to understand, such as obtaining new insights into how employees can work more effectively.
Enterprise leaders don’t need to understand the technical details to devise the best implementation strategy, but they do need to make change management a priority to obtain the needed buy-in from employees. In addition, an agile approach will help leaders realize their vision more quickly.
Prioritizing processes
Most organizations will find prioritizing processes to be their greatest challenge in implementing process intelligence. These solutions typically provide the greatest benefits for accounting and finance departments, primarily due to the likelihood of these areas running legacy systems.
Third-party service providers often provide insight into the best practices for prioritizing workflows with the greatest need for improvement.
Real-world examples of process intelligence implementation
Common use cases for process intelligence include procurement, HR, and IT.
Procurement
The greatest benefits of process intelligence in procurement include end-to-end visibility into processes, especially for detecting bottlenecks. This achievement requires you to map the current processes, collaborate with the relevant teams, and align the new processes with best practices and larger goals like profit generation and cost-savings.
Prioritize the reduction of lead time for core processes, which will typically involve eliminating manual work like free-text approvals. Process intelligence can also help ensure that payments are made on time.
HR
The most useful applications for process intelligence include recruitment, with tasks such as handling candidate applications, interviews, offers, and acceptances. All these steps require data that process mining can help HR departments manage.
Process intelligence can also generate electronic documents that require approval from multiple parties, which helps shorten the hiring process.
IT
Process intelligence in IT can reduce the maintenance costs for enterprise software like ERP by identifying capability gaps. It can also improve the chances of implementing a good solution on the first try through the use of process mining tools that deliver data-driven insights.
In addition, process mining can help companies discover the root cause of delays in resolving long-running tickets.
Future trends for process intelligence
The role of AI and ML is one of the most significant upcoming developments in process intelligence. These technologies will be a source of greater investment and innovation that provide process intelligence with more ways to capture and enhance the expertise of the workforce.
This trend will also increase the adoption of intelligent process automation, especially for enterprises.
Build intelligent and efficient processes with Pipefy
Reduce the time processes spend in limbo between approvals with easy-to-build automations and overdue alerts. Pipefy’s no-code BPA solution offers a range of tools for optimizing workflows from a centralized interface — no coding experience or lengthy IT backlogs required.
With automated alerts, seamlessly integrated systems, and customizable performance dashboards and reporting, tap into powerful process intelligence solutions with total visibility and control over processes, communication, and data.
Looking to add an artificial intelligence tool to your tech stack? We’re thrilled to announce the launch of Pipefy AI. Get quick, accurate answers to questions, or simply tell it what you need, and watch it build processes and strategic insights or suggestions for you.
FAQ about process intelligence
What is the difference between process intelligence and process mining?
As explained in this article, process mining is a component of process intelligence. While process mining focuses on analyzing event logs to discover and visualize process flows, process intelligence encompasses a wider range of activities.
What is process intelligence in BPM?
Process intelligence in Business Process Management (BPM) refers to the ability to gather, analyze, and interpret data from various processes within an organization. It involves using advanced analytics and tools to gain insights into how processes are performed, identifying bottlenecks, inefficiencies, and opportunities for improvement.
What is process intelligence automation?
Process intelligence automation refers to the integration of process intelligence capabilities with automation technologies. It combines the analysis and insights provided by process intelligence with automation tools such as Robotic Process Automation (RPA) and workflow automation. This synergy allows organizations to not only understand their processes but also to automate and optimize them based on data-driven insights.